The End of Brute Force AI: A New Era of Reasoning and Intelligence (Meta-description: AI, Artificial Intelligence, Deep Learning, Reasoning, Pre-training, Ilya Sutskever, NeurIPS, Future of AI)
Imagine a world where AI isn't just a sophisticated parrot, mimicking patterns and spitting out pre-programmed responses, but a true intellectual peer, capable of genuine reasoning and nuanced understanding. This isn't science fiction; it's the future envisioned by Ilya Sutskever, OpenAI's co-founder and former chief scientist. His recent declaration at NeurIPS 2023, that the era of "brute-force" pre-training is over, sent shockwaves through the AI community. This isn't a subtle shift; it's a paradigm change, akin to the transition from the abacus to the digital computer. For years, we've been throwing mountains of data and computational power at AI models, hoping for emergent intelligence. This "throw-it-at-the-wall-and-see-what-sticks" approach has yielded impressive results – stunning image generation, eloquent text synthesis, even game-playing prowess that rivals human champions. But Sutskever's pronouncement signals a fundamental rethinking. We're moving beyond the limitations of sheer scale, embracing a more sophisticated, human-like approach focused on reasoning and critical thinking. Think about it – how often do you solve problems simply by throwing data at them? You use logic, intuition, and prior knowledge. The future of AI, Sutskever implies, demands the same. This isn't just about incremental improvements; it’s a revolution poised to reshape industries, redefine our understanding of intelligence, and usher in a new era of unprecedented technological advancement. Are you ready to delve into this exciting new frontier? Let's explore the implications of this seismic shift and what it means for the future of AI.
The Demise of Pre-training: A Paradigm Shift in AI Development
For years, the dominant paradigm in AI development has been "pre-training." This involved feeding massive datasets into enormous neural networks, hoping they'd learn underlying patterns and relationships. Think of it as teaching a child by inundating them with flashcards – effective, to an extent, but limited in its capacity to foster genuine understanding. While this method delivered impressive results – think of impressive language models like GPT-3 – it's inherently limited. The models, while adept at pattern recognition, often lack the ability to reason, to connect disparate pieces of information, or to adapt to novel situations. They're essentially sophisticated pattern-matching machines, not genuinely intelligent entities. Sutskever's statement signals a decisive move away from this brute-force approach. He argues that future AI will need to move beyond the reliance on sheer scale and embrace more sophisticated reasoning capabilities. This requires a fundamental shift in the way we develop and train AI models. We need to move beyond simply throwing data at the problem.
This isn't to diminish the achievements of pre-training. It's been a crucial stepping stone, providing the foundation upon which future advancements will be built. However, Sutskever's insightful observation highlights that we've reached a point of diminishing returns. Simply scaling up pre-training models further will likely yield diminishing returns; true progress requires a fundamental change in approach. This shift is not unlike the evolution of computing itself. Initially, raw computing power was the primary focus. Then, as technology advanced, the emphasis shifted to efficient algorithms and software optimization. Similarly, in AI, the focus is shifting from sheer scale to sophisticated algorithms that enable reasoning and problem-solving.
The Future of AI: Reasoning, Not Just Recall
So, what does this new era of AI look like? Sutskever hints at a future where AI systems possess a greater capacity for reasoning and inference. Imagine AI that can not only process information but also understand context, draw logical conclusions, and even explain its own reasoning process. This isn't a futuristic fantasy; it's a tangible goal. The shift towards reasoning-centric AI will require new architectures, training methodologies, and evaluation metrics. The focus will be on building models that can not only learn from data but also reason about it, adapt to new situations, and generalize knowledge.
This transition requires a shift in our evaluation metrics. Benchmarking AI models solely on their performance on specific tasks is no longer sufficient. We need to assess their ability to reason, to solve problems creatively, and to adapt to unforeseen circumstances. Developing robust evaluation methods is therefore crucial for guiding the development of truly intelligent AI systems. Furthermore, explaining AI’s reasoning process will become paramount, moving beyond the "black box" nature of many current models. This increased transparency is crucial for building trust and ensuring responsible use.
The development of explainable AI (XAI) is absolutely critical. As AI systems become more complex and powerful, understanding how they arrive at their conclusions becomes increasingly important. XAI aims to shed light on the internal workings of AI models, making their decision-making processes more transparent and understandable. This is not only crucial for debugging and improving AI models but also for building trust and ensuring responsible AI development.
Challenges and Opportunities: Navigating the New Landscape
The transition to reasoning-centric AI is not without its challenges. Developing AI models capable of sophisticated reasoning is a complex undertaking, requiring significant advancements in both algorithms and hardware. Moreover, the evaluation of these models requires new approaches that go beyond simple accuracy metrics. However, the potential benefits are immense. While the path forward is not without its difficulties, the potential rewards are significant.
The economic implications are potentially transformative. Reasoning-centric AI could revolutionize industries ranging from healthcare and finance to manufacturing and transportation. Imagine AI systems that can diagnose diseases with greater accuracy, develop personalized treatment plans, or optimize supply chains with unprecedented efficiency. Furthermore, the societal impact could be profound. AI systems capable of genuine reasoning could help us address some of the world’s most pressing challenges, from climate change to poverty.
However, alongside these transformative opportunities come ethical considerations that are critical to address. The development and deployment of reasoning-centric AI must be guided by ethical principles, and robust mechanisms are needed to ensure responsible innovation and prevent misuse. We must grapple with questions of bias, fairness, accountability, and transparency. Open dialogue and collaboration between researchers, policymakers, and the public are essential to guide the development of AI in a way that benefits humanity.
FAQs: Addressing Your Burning Questions
Here are some common questions regarding this AI paradigm shift:
Q1: What exactly is “brute-force” pre-training?
A1: It refers to training AI models using massive datasets and immense computational power, relying on sheer scale rather than sophisticated algorithms for learning. Think of it as throwing data at a problem until something works.
Q2: Will pre-trained models become obsolete?
A2: Not entirely. Pre-training will likely remain a valuable component of AI development, but its role will shift. It will serve as a foundation upon which reasoning capabilities are built, rather than being the primary engine of intelligence.
Q3: How will reasoning be implemented in AI?
A3: This is an active area of research. Potential approaches include incorporating symbolic reasoning techniques into neural networks, developing more sophisticated architectures that can handle complex relationships, and employing techniques that allow AI to learn and reason from fewer data points.
Q4: What are the ethical implications of this shift?
A4: Reasoning-centric AI raises important ethical questions regarding bias, fairness, accountability, and transparency. Ensuring responsible development and deployment requires careful consideration of these issues.
Q5: How long will it take to see widespread adoption of reasoning-centric AI?
A5: This is difficult to predict, but the shift is likely to be gradual. We can expect incremental progress in the coming years, with wider adoption following significant advancements in algorithms and hardware.
Q6: Will this new approach make AI more expensive to develop?
A6: Potentially, yes, at least initially. Developing sophisticated reasoning capabilities will likely require more advanced algorithms and hardware. However, the long-term benefits—in efficiency and capability—could offset these initial costs.
Conclusion: A New Dawn for Artificial Intelligence
The end of the "brute-force" era of AI marks a pivotal moment in the history of artificial intelligence. Ilya Sutskever’s insightful pronouncement signals a fundamental shift away from simply scaling up models and towards cultivating true reasoning abilities. This isn't merely about incremental improvements; it's a revolutionary leap towards creating AI systems that are not only powerful but also intelligent, adaptable, and capable of genuine problem-solving. While challenges remain, the potential benefits are immense, promising a future where AI plays an increasingly transformative role in addressing global challenges and enhancing human capabilities. The journey towards reasoning-centric AI is filled with both excitement and uncertainty, but one thing is certain: the future of AI is bright, and the future is now.
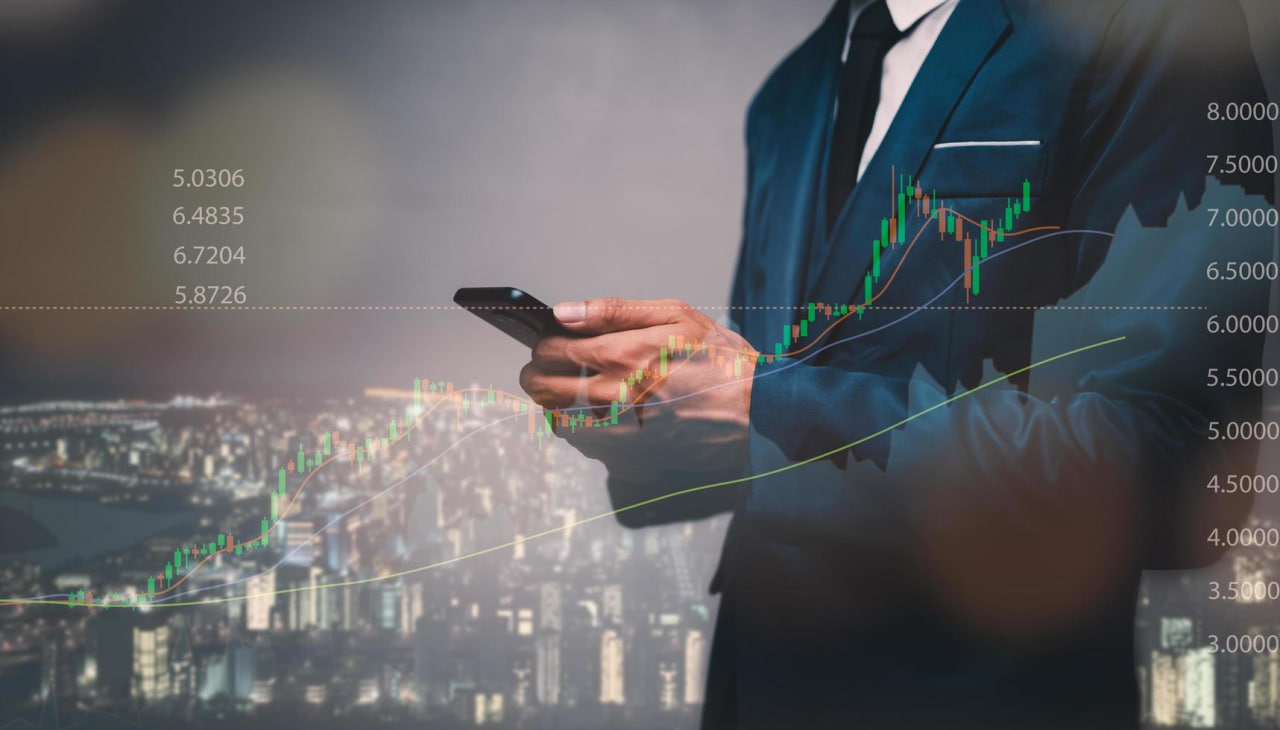